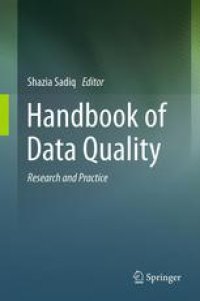
Ebook: Handbook of Data Quality: Research and Practice
- Genre: Computers // Algorithms and Data Structures
- Tags: Database Management, Information Storage and Retrieval, Management of Computing and Information Systems, Files
- Year: 2013
- Publisher: Springer-Verlag Berlin Heidelberg
- Edition: 1
- Language: English
- pdf
The issue of data quality is as old as data itself. However, the proliferation of diverse, large-scale and often publically available data on the Web has increased the risk of poor data quality and misleading data interpretations. On the other hand, data is now exposed at a much more strategic level e.g. through business intelligence systems, increasing manifold the stakes involved for individuals, corporations as well as government agencies. There, the lack of knowledge about data accuracy, currency or completeness can have erroneous and even catastrophic results.
With these changes, traditional approaches to data management in general, and data quality control specifically, are challenged. There is an evident need to incorporate data quality considerations into the whole data cycle, encompassing managerial/governance as well as technical aspects.
Data quality experts from research and industry agree that a unified framework for data quality management should bring together organizational, architectural and computational approaches. Accordingly, Sadiq structured this handbook in four parts: Part I is on organizational solutions, i.e. the development of data quality objectives for the organization, and the development of strategies to establish roles, processes, policies, and standards required to manage and ensure data quality. Part II, on architecturalsolutions, covers the technology landscape required to deploy developed data quality management processes, standards and policies. Part III, on computationalsolutions, presents effective and efficient tools and techniques related to record linkage, lineage and provenance, data uncertainty, and advanced integrity constraints. Finally, Part IV is devoted to case studies of successful data quality initiatives that highlight the various aspects of data quality in action. The individual chapters present both an overview of the respective topic in terms of historical research and/or practice and state of the art, as well as specific techniques, methodologies and frameworks developed by the individual contributors.
Researchers and students of computer science, information systems, or business management as well as data professionals and practitioners will benefit most from this handbook by not only focusing on the various sections relevant to their research area or particular practical work, but by also studying chapters that they may initially consider not to be directly relevant to them, as there they will learn about new perspectives and approaches.
The issue of data quality is as old as data itself. However, the proliferation of diverse, large-scale and often publically available data on the Web has increased the risk of poor data quality and misleading data interpretations. On the other hand, data is now exposed at a much more strategic level e.g. through business intelligence systems, increasing manifold the stakes involved for individuals, corporations as well as government agencies. There, the lack of knowledge about data accuracy, currency or completeness can have erroneous and even catastrophic results.
With these changes, traditional approaches to data management in general, and data quality control specifically, are challenged. There is an evident need to incorporate data quality considerations into the whole data cycle, encompassing managerial/governance as well as technical aspects.
Data quality experts from research and industry agree that a unified framework for data quality management should bring together organizational, architectural and computational approaches. Accordingly, Sadiq structured this handbook in four parts: Part I is on organizational solutions, i.e. the development of data quality objectives for the organization, and the development of strategies to establish roles, processes, policies, and standards required to manage and ensure data quality. Part II, on architecturalsolutions, covers the technology landscape required to deploy developed data quality management processes, standards and policies. Part III, on computationalsolutions, presents effective and efficient tools and techniques related to record linkage, lineage and provenance, data uncertainty, and advanced integrity constraints. Finally, Part IV is devoted to case studies of successful data quality initiatives that highlight the various aspects of data quality in action. The individual chapters present both an overview of the respective topic in terms of historical research and/or practice and state of the art, as well as specific techniques, methodologies and frameworks developed by the individual contributors.
Researchers and students of computer science, information systems, or business management as well as data professionals and practitioners will benefit most from this handbook by not only focusing on the various sections relevant to their research area or particular practical work, but by also studying chapters that they may initially consider not to be directly relevant to them, as there they will learn about new perspectives and approaches.
The issue of data quality is as old as data itself. However, the proliferation of diverse, large-scale and often publically available data on the Web has increased the risk of poor data quality and misleading data interpretations. On the other hand, data is now exposed at a much more strategic level e.g. through business intelligence systems, increasing manifold the stakes involved for individuals, corporations as well as government agencies. There, the lack of knowledge about data accuracy, currency or completeness can have erroneous and even catastrophic results.
With these changes, traditional approaches to data management in general, and data quality control specifically, are challenged. There is an evident need to incorporate data quality considerations into the whole data cycle, encompassing managerial/governance as well as technical aspects.
Data quality experts from research and industry agree that a unified framework for data quality management should bring together organizational, architectural and computational approaches. Accordingly, Sadiq structured this handbook in four parts: Part I is on organizational solutions, i.e. the development of data quality objectives for the organization, and the development of strategies to establish roles, processes, policies, and standards required to manage and ensure data quality. Part II, on architecturalsolutions, covers the technology landscape required to deploy developed data quality management processes, standards and policies. Part III, on computationalsolutions, presents effective and efficient tools and techniques related to record linkage, lineage and provenance, data uncertainty, and advanced integrity constraints. Finally, Part IV is devoted to case studies of successful data quality initiatives that highlight the various aspects of data quality in action. The individual chapters present both an overview of the respective topic in terms of historical research and/or practice and state of the art, as well as specific techniques, methodologies and frameworks developed by the individual contributors.
Researchers and students of computer science, information systems, or business management as well as data professionals and practitioners will benefit most from this handbook by not only focusing on the various sections relevant to their research area or particular practical work, but by also studying chapters that they may initially consider not to be directly relevant to them, as there they will learn about new perspectives and approaches.
Content:
Front Matter....Pages i-xii
Front Matter....Pages 13-14
Data Quality Management Past, Present, and Future: Towards a Management System for Data....Pages 15-40
Data Quality Projects and Programs....Pages 41-73
Cost and Value Management for Data Quality....Pages 75-92
On the Evolution of Data Governance in Firms: The Case of Johnson & Johnson Consumer Products North America....Pages 93-118
Front Matter....Pages 119-119
Data Warehouse Quality: Summary and Outlook....Pages 121-140
Using Semantic Web Technologies for Data Quality Management....Pages 141-161
Data Glitches: Monsters in Your Data....Pages 163-178
Front Matter....Pages 179-180
Generic and Declarative Approaches to Data Quality Management....Pages 181-211
Linking Records in Complex Context....Pages 213-233
A Practical Guide to Entity Resolution with OYSTER....Pages 235-270
Managing Quality of Probabilistic Databases....Pages 271-291
Data Fusion: Resolving Conflicts from Multiple Sources....Pages 293-318
Front Matter....Pages 319-319
Ensuring the Quality of Health Information: The Canadian Experience....Pages 321-346
Shell’s Global Data Quality Journey....Pages 347-368
Creating an Information-Centric Organisation Culture at SBI General Insurance....Pages 369-395
Prologue: Research and Practice in Data Quality Management....Pages 1-11
Epilogue: The Data Quality Profession....Pages 397-417
Back Matter....Pages 419-438