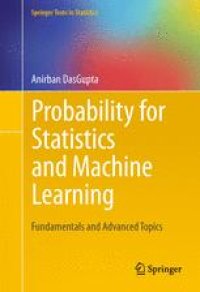
Ebook: Probability for Statistics and Machine Learning: Fundamentals and Advanced Topics
Author: Anirban DasGupta (auth.)
- Tags: Statistical Theory and Methods, Probability Theory and Stochastic Processes, Simulation and Modeling, Bioinformatics
- Series: Springer Texts in Statistics
- Year: 2011
- Publisher: Springer-Verlag New York
- Edition: 1
- Language: English
- pdf
This book provides a versatile and lucid treatment of classic as well as modern probability theory, while integrating them with core topics in statistical theory and also some key tools in machine learning. It is written in an extremely accessible style, with elaborate motivating discussions and numerous worked out examples and exercises. The book has 20 chapters on a wide range of topics, 423 worked out examples, and 808 exercises. It is unique in its unification of probability and statistics, its coverage and its superb exercise sets, detailed bibliography, and in its substantive treatment of many topics of current importance.
This book can be used as a text for a year long graduate course in statistics, computer science, or mathematics, for self-study, and as an invaluable research reference on probabiliity and its applications. Particularly worth mentioning are the treatments of distribution theory, asymptotics, simulation and Markov Chain Monte Carlo, Markov chains and martingales, Gaussian processes, VC theory, probability metrics, large deviations, bootstrap, the EM algorithm, confidence intervals, maximum likelihood and Bayes estimates, exponential families, kernels, and Hilbert spaces, and a self contained complete review of univariate probability.
This book provides a versatile and lucid treatment of classic as well as modern probability theory, while integrating them with core topics in statistical theory and also some key tools in machine learning. It is written in an extremely accessible style, with elaborate motivating discussions and numerous worked out examples and exercises. The book has 20 chapters on a wide range of topics, 423 worked out examples, and 808 exercises. It is unique in its unification of probability and statistics, its coverage and its superb exercise sets, detailed bibliography, and in its substantive treatment of many topics of current importance.
This book can be used as a text for a year long graduate course in statistics, computer science, or mathematics, for self-study, and as an invaluable research reference on probabiliity and its applications. Particularly worth mentioning are the treatments of distribution theory, asymptotics, simulation and Markov Chain Monte Carlo, Markov chains and martingales, Gaussian processes, VC theory, probability metrics, large deviations, bootstrap, the EM algorithm, confidence intervals, maximum likelihood and Bayes estimates, exponential families, kernels, and Hilbert spaces, and a self contained complete review of univariate probability.
This book provides a versatile and lucid treatment of classic as well as modern probability theory, while integrating them with core topics in statistical theory and also some key tools in machine learning. It is written in an extremely accessible style, with elaborate motivating discussions and numerous worked out examples and exercises. The book has 20 chapters on a wide range of topics, 423 worked out examples, and 808 exercises. It is unique in its unification of probability and statistics, its coverage and its superb exercise sets, detailed bibliography, and in its substantive treatment of many topics of current importance.
This book can be used as a text for a year long graduate course in statistics, computer science, or mathematics, for self-study, and as an invaluable research reference on probabiliity and its applications. Particularly worth mentioning are the treatments of distribution theory, asymptotics, simulation and Markov Chain Monte Carlo, Markov chains and martingales, Gaussian processes, VC theory, probability metrics, large deviations, bootstrap, the EM algorithm, confidence intervals, maximum likelihood and Bayes estimates, exponential families, kernels, and Hilbert spaces, and a self contained complete review of univariate probability.
Content:
Front Matter....Pages i-xix
Review of Univariate Probability....Pages 1-93
Multivariate Discrete Distributions....Pages 95-121
Multidimensional Densities....Pages 123-165
Advanced Distribution Theory....Pages 167-197
Multivariate Normal and Related Distributions....Pages 199-219
Finite Sample Theory of Order Statistics and Extremes....Pages 221-248
Essential Asymptotics and Applications....Pages 249-292
Characteristic Functions and Applications....Pages 293-322
Asymptotics of Extremes and Order Statistics....Pages 323-338
Markov Chains and Applications....Pages 339-374
Random Walks....Pages 375-400
Brownian Motion and Gaussian Processes....Pages 401-436
Poisson Processes and Applications....Pages 437-462
Discrete Time Martingales and Concentration Inequalities....Pages 463-504
Probability Metrics....Pages 505-526
Empirical Processes and VC Theory....Pages 527-558
Large Deviations....Pages 559-582
The Exponential Family and Statistical Applications....Pages 583-612
Simulation and Markov Chain Monte Carlo....Pages 613-687
Useful Tools for Statistics and Machine Learning....Pages 689-746
Back Matter....Pages 747-782
This book provides a versatile and lucid treatment of classic as well as modern probability theory, while integrating them with core topics in statistical theory and also some key tools in machine learning. It is written in an extremely accessible style, with elaborate motivating discussions and numerous worked out examples and exercises. The book has 20 chapters on a wide range of topics, 423 worked out examples, and 808 exercises. It is unique in its unification of probability and statistics, its coverage and its superb exercise sets, detailed bibliography, and in its substantive treatment of many topics of current importance.
This book can be used as a text for a year long graduate course in statistics, computer science, or mathematics, for self-study, and as an invaluable research reference on probabiliity and its applications. Particularly worth mentioning are the treatments of distribution theory, asymptotics, simulation and Markov Chain Monte Carlo, Markov chains and martingales, Gaussian processes, VC theory, probability metrics, large deviations, bootstrap, the EM algorithm, confidence intervals, maximum likelihood and Bayes estimates, exponential families, kernels, and Hilbert spaces, and a self contained complete review of univariate probability.
Content:
Front Matter....Pages i-xix
Review of Univariate Probability....Pages 1-93
Multivariate Discrete Distributions....Pages 95-121
Multidimensional Densities....Pages 123-165
Advanced Distribution Theory....Pages 167-197
Multivariate Normal and Related Distributions....Pages 199-219
Finite Sample Theory of Order Statistics and Extremes....Pages 221-248
Essential Asymptotics and Applications....Pages 249-292
Characteristic Functions and Applications....Pages 293-322
Asymptotics of Extremes and Order Statistics....Pages 323-338
Markov Chains and Applications....Pages 339-374
Random Walks....Pages 375-400
Brownian Motion and Gaussian Processes....Pages 401-436
Poisson Processes and Applications....Pages 437-462
Discrete Time Martingales and Concentration Inequalities....Pages 463-504
Probability Metrics....Pages 505-526
Empirical Processes and VC Theory....Pages 527-558
Large Deviations....Pages 559-582
The Exponential Family and Statistical Applications....Pages 583-612
Simulation and Markov Chain Monte Carlo....Pages 613-687
Useful Tools for Statistics and Machine Learning....Pages 689-746
Back Matter....Pages 747-782
....