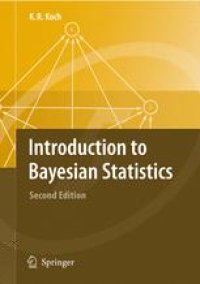
Ebook: Introduction to Bayesian Statistics
- Tags: Geophysics/Geodesy, Statistics for Engineering Physics Computer Science Chemistry & Geosciences, Geographical Information Systems/Cartography, Image Processing and Computer Vision
- Year: 2007
- Publisher: Springer-Verlag Berlin Heidelberg
- Edition: 2
- Language: English
- pdf
From the reviews of the second edition:
"This is a well-written introduction to Bayesian Analysis that contains many applications to Geodesy and Engineering at the cutting edge of these topics. … There is a good treatment of Bayesian Analysis of Linear Models … . The references are very interesting … by a group of scientists of whose work many of us in the Statistical profession may not be aware. The strength of the book lies in its coverage, careful mathematics and many contemporary applications." (Jayanta K. Ghosh, International Statistical Review, Vol. 76 (1), 2008)
The Introduction to Bayesian Statistics (2nd edition) presents Bayes’ theorem, the estimation of unknown parameters, the determination of confidence regions and the derivation of tests of hypotheses for the unknown parameters. It does so in a manner that is simple, intuitive and easy to comprehend.
The methods are applied to linear models, in models for a robust estimation, for prediction and filtering and in models for estimating variance components and covariance components. Regularization of inverse problems and pattern recognition are also covered while Bayesian networks serve for reaching decisions in systems with uncertainties. If analytical solutions cannot be derived, numerical algorithms are presented, such as the Monte Carlo integration and Markov Chain Monte Carlo methods.
The Introduction to Bayesian Statistics (2nd edition) presents Bayes’ theorem, the estimation of unknown parameters, the determination of confidence regions and the derivation of tests of hypotheses for the unknown parameters. It does so in a manner that is simple, intuitive and easy to comprehend.
The methods are applied to linear models, in models for a robust estimation, for prediction and filtering and in models for estimating variance components and covariance components. Regularization of inverse problems and pattern recognition are also covered while Bayesian networks serve for reaching decisions in systems with uncertainties. If analytical solutions cannot be derived, numerical algorithms are presented, such as the Monte Carlo integration and Markov Chain Monte Carlo methods.
Content:
Front Matter....Pages I-XII
Introduction....Pages 1-1
Probability....Pages 3-62
Parameter Estimation, Confidence Regions and Hypothesis Testing....Pages 63-83
Linear Model....Pages 85-127
Special Models and Applications....Pages 129-192
Numerical Methods....Pages 193-234
Back Matter....Pages 235-249
The Introduction to Bayesian Statistics (2nd edition) presents Bayes’ theorem, the estimation of unknown parameters, the determination of confidence regions and the derivation of tests of hypotheses for the unknown parameters. It does so in a manner that is simple, intuitive and easy to comprehend.
The methods are applied to linear models, in models for a robust estimation, for prediction and filtering and in models for estimating variance components and covariance components. Regularization of inverse problems and pattern recognition are also covered while Bayesian networks serve for reaching decisions in systems with uncertainties. If analytical solutions cannot be derived, numerical algorithms are presented, such as the Monte Carlo integration and Markov Chain Monte Carlo methods.
Content:
Front Matter....Pages I-XII
Introduction....Pages 1-1
Probability....Pages 3-62
Parameter Estimation, Confidence Regions and Hypothesis Testing....Pages 63-83
Linear Model....Pages 85-127
Special Models and Applications....Pages 129-192
Numerical Methods....Pages 193-234
Back Matter....Pages 235-249
....