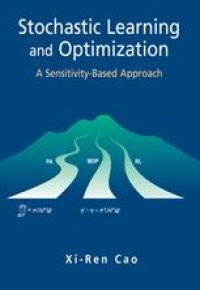
Ebook: Stochastic Learning and Optimization: A Sensitivity-Based Approach
Author: Xi-Ren Cao PhD (auth.)
- Tags: Discrete Mathematics in Computer Science, Artificial Intelligence (incl. Robotics), Calculus of Variations and Optimal Control Optimization, Probability Theory and Stochastic Processes, Operations Research/Decision Theory
- Year: 2007
- Publisher: Springer US
- Edition: 1
- Language: English
- pdf
Stochastic learning and optimization is a multidisciplinary subject that has wide applications in modern engineering, social, and financial problems, including those in Internet and wireless communications, manufacturing, robotics, logistics, biomedical systems, and investment science. This book is unique in the following aspects.
- (Four areas in one book) This book covers various disciplines in learning and optimization, including perturbation analysis (PA) of discrete-event dynamic systems, Markov decision processes (MDP)s), reinforcement learning (RL), and adaptive control, within a unified framework.
- (A simple approach to MDPs) This book introduces MDP theory through a simple approach based on performance difference formulas. This approach leads to results for the n-bias optimality with long-run average-cost criteria and Blackwell's optimality without discounting.
- (Event-based optimization) This book introduces the recently developed event-based optimization approach, which opens up a research direction in overcoming or alleviating the difficulties due to the curse of dimensionality issue by utilizing the system's special features.
- (Sample-path construction) This book emphasizes physical interpretations based on the sample-path construction.
Stochastic learning and optimization is a multidisciplinary subject that has wide applications in modern engineering, social, and financial problems, including those in Internet and wireless communications, manufacturing, robotics, logistics, biomedical systems, and investment science. This book is unique in the following aspects.
- (Four areas in one book) This book covers various disciplines in learning and optimization, including perturbation analysis (PA) of discrete-event dynamic systems, Markov decision processes (MDP)s), reinforcement learning (RL), and adaptive control, within a unified framework.
- (A simple approach to MDPs) This book introduces MDP theory through a simple approach based on performance difference formulas. This approach leads to results for the n-bias optimality with long-run average-cost criteria and Blackwell's optimality without discounting.
- (Event-based optimization) This book introduces the recently developed event-based optimization approach, which opens up a research direction in overcoming or alleviating the difficulties due to the curse of dimensionality issue by utilizing the system's special features.
- (Sample-path construction) This book emphasizes physical interpretations based on the sample-path construction.
Stochastic learning and optimization is a multidisciplinary subject that has wide applications in modern engineering, social, and financial problems, including those in Internet and wireless communications, manufacturing, robotics, logistics, biomedical systems, and investment science. This book is unique in the following aspects.
- (Four areas in one book) This book covers various disciplines in learning and optimization, including perturbation analysis (PA) of discrete-event dynamic systems, Markov decision processes (MDP)s), reinforcement learning (RL), and adaptive control, within a unified framework.
- (A simple approach to MDPs) This book introduces MDP theory through a simple approach based on performance difference formulas. This approach leads to results for the n-bias optimality with long-run average-cost criteria and Blackwell's optimality without discounting.
- (Event-based optimization) This book introduces the recently developed event-based optimization approach, which opens up a research direction in overcoming or alleviating the difficulties due to the curse of dimensionality issue by utilizing the system's special features.
- (Sample-path construction) This book emphasizes physical interpretations based on the sample-path construction.
Content:
Front Matter....Pages I-XIX
Front Matter....Pages 1-1
Introduction....Pages 1-48
Front Matter....Pages 49-49
Perturbation Analysis....Pages 51-146
Learning and Optimization with Perturbation Analysis....Pages 147-182
Markov Decision Processes....Pages 183-252
Sample-Path-Based Policy Iteration....Pages 253-287
Reinforcement Learning....Pages 289-340
Adaptive Control Problems as MDPs....Pages 341-383
Front Matter....Pages 385-385
Event-Based Optimization of Markov Systems....Pages 387-454
Constructing Sensitivity Formulas....Pages 455-486
Back Matter....Pages 489-566
Stochastic learning and optimization is a multidisciplinary subject that has wide applications in modern engineering, social, and financial problems, including those in Internet and wireless communications, manufacturing, robotics, logistics, biomedical systems, and investment science. This book is unique in the following aspects.
- (Four areas in one book) This book covers various disciplines in learning and optimization, including perturbation analysis (PA) of discrete-event dynamic systems, Markov decision processes (MDP)s), reinforcement learning (RL), and adaptive control, within a unified framework.
- (A simple approach to MDPs) This book introduces MDP theory through a simple approach based on performance difference formulas. This approach leads to results for the n-bias optimality with long-run average-cost criteria and Blackwell's optimality without discounting.
- (Event-based optimization) This book introduces the recently developed event-based optimization approach, which opens up a research direction in overcoming or alleviating the difficulties due to the curse of dimensionality issue by utilizing the system's special features.
- (Sample-path construction) This book emphasizes physical interpretations based on the sample-path construction.
Content:
Front Matter....Pages I-XIX
Front Matter....Pages 1-1
Introduction....Pages 1-48
Front Matter....Pages 49-49
Perturbation Analysis....Pages 51-146
Learning and Optimization with Perturbation Analysis....Pages 147-182
Markov Decision Processes....Pages 183-252
Sample-Path-Based Policy Iteration....Pages 253-287
Reinforcement Learning....Pages 289-340
Adaptive Control Problems as MDPs....Pages 341-383
Front Matter....Pages 385-385
Event-Based Optimization of Markov Systems....Pages 387-454
Constructing Sensitivity Formulas....Pages 455-486
Back Matter....Pages 489-566
....