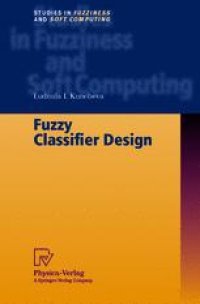
Ebook: Fuzzy Classifier Design
Author: Dr. Ludmila I. Kuncheva (auth.)
- Tags: Artificial Intelligence (incl. Robotics), Pattern Recognition, Business Information Systems
- Series: Studies in Fuzziness and Soft Computing 49
- Year: 2000
- Publisher: Physica-Verlag Heidelberg
- Edition: 1
- Language: English
- pdf
Fuzzy sets were first proposed by Lotfi Zadeh in his seminal paper [366] in 1965, and ever since have been a center of many discussions, fervently admired and condemned. Both proponents and opponents consider the argu ments pointless because none of them would step back from their territory. And stiH, discussions burst out from a single sparkle like a conference pa per or a message on some fuzzy-mail newsgroup. Here is an excerpt from an e-mail messagepostedin1993tofuzzy-mail@vexpert. dbai. twvien. ac. at. by somebody who signed "Dave". , . . . Why then the "logic" in "fuzzy logic"? I don't think anyone has successfully used fuzzy sets for logical inference, nor do I think anyone wiH. In my admittedly neophyte opinion, "fuzzy logic" is a misnomer, an oxymoron. (1 would be delighted to be proven wrong on that. ) . . . I carne to the fuzzy literature with an open mind (and open wal let), high hopes and keen interest. I am very much disiHusioned with "fuzzy" per se, but I did happen across some extremely interesting things along the way. " Dave, thanks for the nice quote! Enthusiastic on the surface, are not many of us suspicious deep down? In some books and journals the word fuzzy is religiously avoided: fuzzy set theory is viewed as a second-hand cheap trick whose aim is nothing else but to devalue good classical theories and open up the way to lazy ignorants and newcomers.
This book about fuzzy classifier design briefly introduces the fundamentals of supervised pattern recognition and fuzzy set theory. Fuzzy if-then classifiers are defined and some theoretical properties thereof are studied. Popular training algorithms are detailed. Non if-then fuzzy classifiers include relational, k-nearest neighbor, prototype-based designs, etc. A chapter on multiple classifier combination discusses fuzzy and non-fuzzy models for fusion and selection.
This book about fuzzy classifier design briefly introduces the fundamentals of supervised pattern recognition and fuzzy set theory. Fuzzy if-then classifiers are defined and some theoretical properties thereof are studied. Popular training algorithms are detailed. Non if-then fuzzy classifiers include relational, k-nearest neighbor, prototype-based designs, etc. A chapter on multiple classifier combination discusses fuzzy and non-fuzzy models for fusion and selection.
Content:
Front Matter....Pages i-x
Introduction....Pages 1-13
Statistical pattern recognition....Pages 15-36
Statistical classifiers....Pages 37-78
Fuzzy sets....Pages 79-115
Fuzzy if-then classifiers....Pages 117-155
Training of fuzzy if-then classifiers....Pages 157-196
Non if-then fuzzy models....Pages 197-231
Combinations of multiple classifiers using fuzzy sets....Pages 233-267
Conclusions: What to choose?....Pages 269-270
Back Matter....Pages 271-315
This book about fuzzy classifier design briefly introduces the fundamentals of supervised pattern recognition and fuzzy set theory. Fuzzy if-then classifiers are defined and some theoretical properties thereof are studied. Popular training algorithms are detailed. Non if-then fuzzy classifiers include relational, k-nearest neighbor, prototype-based designs, etc. A chapter on multiple classifier combination discusses fuzzy and non-fuzzy models for fusion and selection.
Content:
Front Matter....Pages i-x
Introduction....Pages 1-13
Statistical pattern recognition....Pages 15-36
Statistical classifiers....Pages 37-78
Fuzzy sets....Pages 79-115
Fuzzy if-then classifiers....Pages 117-155
Training of fuzzy if-then classifiers....Pages 157-196
Non if-then fuzzy models....Pages 197-231
Combinations of multiple classifiers using fuzzy sets....Pages 233-267
Conclusions: What to choose?....Pages 269-270
Back Matter....Pages 271-315
....