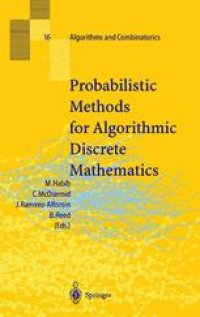
Ebook: Probabilistic Methods for Algorithmic Discrete Mathematics
Author: Michael Molloy (auth.) Michel Habib Colin McDiarmid Jorge Ramirez-Alfonsin Bruce Reed (eds.)
- Tags: Combinatorics, Computation by Abstract Devices, Symbolic and Algebraic Manipulation, Probability Theory and Stochastic Processes
- Series: Algorithms and Combinatorics 16
- Year: 1998
- Publisher: Springer-Verlag Berlin Heidelberg
- Edition: 1
- Language: English
- pdf
The book gives an accessible account of modern pro- babilistic methods for analyzing combinatorial structures and algorithms. Each topic is approached in a didactic manner but the most recent developments are linked to the basic ma- terial. Extensive lists of references and a detailed index will make this a useful guide for graduate students and researchers. Special features included:
- a simple treatment of Talagrand inequalities and their applications
- an overview and many carefully worked out examples of the probabilistic analysis of combinatorial algorithms
- a discussion of the "exact simulation" algorithm (in the context of Markov Chain Monte Carlo Methods)
- a general method for finding asymptotically optimal or near optimal graph colouring, showing how the probabilistic method may be fine-tuned to explit the structure of the underlying graph
- a succinct treatment of randomized algorithms and derandomization techniques
The book gives an accessible account of modern pro- babilistic methods for analyzing combinatorial structures and algorithms. Each topic is approached in a didactic manner but the most recent developments are linked to the basic ma- terial. Extensive lists of references and a detailed index will make this a useful guide for graduate students and researchers. Special features included:
- a simple treatment of Talagrand inequalities and their applications
- an overview and many carefully worked out examples of the probabilistic analysis of combinatorial algorithms
- a discussion of the "exact simulation" algorithm (in the context of Markov Chain Monte Carlo Methods)
- a general method for finding asymptotically optimal or near optimal graph colouring, showing how the probabilistic method may be fine-tuned to explit the structure of the underlying graph
- a succinct treatment of randomized algorithms and derandomization techniques
The book gives an accessible account of modern pro- babilistic methods for analyzing combinatorial structures and algorithms. Each topic is approached in a didactic manner but the most recent developments are linked to the basic ma- terial. Extensive lists of references and a detailed index will make this a useful guide for graduate students and researchers. Special features included:
- a simple treatment of Talagrand inequalities and their applications
- an overview and many carefully worked out examples of the probabilistic analysis of combinatorial algorithms
- a discussion of the "exact simulation" algorithm (in the context of Markov Chain Monte Carlo Methods)
- a general method for finding asymptotically optimal or near optimal graph colouring, showing how the probabilistic method may be fine-tuned to explit the structure of the underlying graph
- a succinct treatment of randomized algorithms and derandomization techniques
Content:
Front Matter....Pages I-XVII
The Probabilistic Method....Pages 1-35
Probabilistic Analysis of Algorithms....Pages 36-92
An Overview of Randomized Algorithms....Pages 93-115
Mathematical Foundations of the Markov Chain Monte Carlo Method....Pages 116-165
Percolation and the Random Cluster Model: Combinatorial and Algorithmic Problems....Pages 166-194
Concentration....Pages 195-248
Branching Processes and Their Applications in the Analysis of Tree Structures and Tree Algorithms....Pages 249-314
Back Matter....Pages 315-325
The book gives an accessible account of modern pro- babilistic methods for analyzing combinatorial structures and algorithms. Each topic is approached in a didactic manner but the most recent developments are linked to the basic ma- terial. Extensive lists of references and a detailed index will make this a useful guide for graduate students and researchers. Special features included:
- a simple treatment of Talagrand inequalities and their applications
- an overview and many carefully worked out examples of the probabilistic analysis of combinatorial algorithms
- a discussion of the "exact simulation" algorithm (in the context of Markov Chain Monte Carlo Methods)
- a general method for finding asymptotically optimal or near optimal graph colouring, showing how the probabilistic method may be fine-tuned to explit the structure of the underlying graph
- a succinct treatment of randomized algorithms and derandomization techniques
Content:
Front Matter....Pages I-XVII
The Probabilistic Method....Pages 1-35
Probabilistic Analysis of Algorithms....Pages 36-92
An Overview of Randomized Algorithms....Pages 93-115
Mathematical Foundations of the Markov Chain Monte Carlo Method....Pages 116-165
Percolation and the Random Cluster Model: Combinatorial and Algorithmic Problems....Pages 166-194
Concentration....Pages 195-248
Branching Processes and Their Applications in the Analysis of Tree Structures and Tree Algorithms....Pages 249-314
Back Matter....Pages 315-325
....