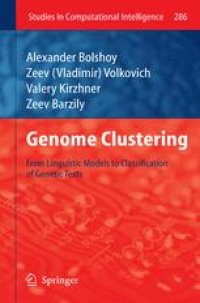
Ebook: Genome Clustering: From Linguistic Models to Classification of Genetic Texts
- Tags: Appl.Mathematics/Computational Methods of Engineering, Artificial Intelligence (incl. Robotics)
- Series: Studies in Computational Intelligence 286
- Year: 2010
- Publisher: Springer-Verlag Berlin Heidelberg
- Edition: 1
- Language: English
- pdf
The study of language texts at the level of formal non-semantic models has a long history. Suffice it to say that the well-known Markov chains were first introduced as one of such models. The representation of biological data as text and, consequently, applications of text-analysis models in the field of comparative genomics are substantially newer; nevertheless the methods are well developed. In this book, we try to juxtapose linguistic and bioinformatics models of text analysis. So, it can be read, in a sense, “in two directions” – the book is written so as to appeal to the bioinformatician, who may be interested in finding techniques that had initially appeared in the natural language analysis, and to computational linguist, who may be surprised to discover familiar methods used in bioinformatics. In the presentation of the material, the authors, nevertheless, give preference their professional field - bioinformatics. Therefore, even a specialist in bioinformatics can find something new himself in this book. For example, this book includes a review of the main data mining models generating the text spectra. The chapters of the book assume neither advanced mathematical skills nor beginner knowledge of molecular biology. Relevant biological concepts are introduced in the beginning of the book. Several computer science issues relevant to the topics of the book are reviewed in the three appendices: clustering, sequence complexity, and DNA curvature modeling.
The study of language texts at the level of formal non-semantic models has a long history. Suffice it to say that the well-known Markov chains were first introduced as one of such models. The representation of biological data as text and, consequently, applications of text-analysis models in the field of comparative genomics are substantially newer; nevertheless the methods are well developed. In this book, we try to juxtapose linguistic and bioinformatics models of text analysis. So, it can be read, in a sense, “in two directions” – the book is written so as to appeal to the bioinformatician, who may be interested in finding techniques that had initially appeared in the natural language analysis, and to computational linguist, who may be surprised to discover familiar methods used in bioinformatics. In the presentation of the material, the authors, nevertheless, give preference their professional field - bioinformatics. Therefore, even a specialist in bioinformatics can find something new himself in this book. For example, this book includes a review of the main data mining models generating the text spectra. The chapters of the book assume neither advanced mathematical skills nor beginner knowledge of molecular biology. Relevant biological concepts are introduced in the beginning of the book. Several computer science issues relevant to the topics of the book are reviewed in the three appendices: clustering, sequence complexity, and DNA curvature modeling.
The study of language texts at the level of formal non-semantic models has a long history. Suffice it to say that the well-known Markov chains were first introduced as one of such models. The representation of biological data as text and, consequently, applications of text-analysis models in the field of comparative genomics are substantially newer; nevertheless the methods are well developed. In this book, we try to juxtapose linguistic and bioinformatics models of text analysis. So, it can be read, in a sense, “in two directions” – the book is written so as to appeal to the bioinformatician, who may be interested in finding techniques that had initially appeared in the natural language analysis, and to computational linguist, who may be surprised to discover familiar methods used in bioinformatics. In the presentation of the material, the authors, nevertheless, give preference their professional field - bioinformatics. Therefore, even a specialist in bioinformatics can find something new himself in this book. For example, this book includes a review of the main data mining models generating the text spectra. The chapters of the book assume neither advanced mathematical skills nor beginner knowledge of molecular biology. Relevant biological concepts are introduced in the beginning of the book. Several computer science issues relevant to the topics of the book are reviewed in the three appendices: clustering, sequence complexity, and DNA curvature modeling.
Content:
Front Matter....Pages -
Biological Background....Pages 1-16
Biological Classification....Pages 17-22
Mathematical Models for the Analysis of Natural-Language Documents....Pages 23-42
DNA Texts....Pages 43-60
N-Gram Spectra of the DNA Text....Pages 61-85
Application of Compositional Spectra to DNA Sequences....Pages 87-112
Marker-Function Profile-Based Clustering....Pages 113-145
Genome as a Bag of Genes – The Whole-Genome Phylogenetics....Pages 147-160
Back Matter....Pages -
The study of language texts at the level of formal non-semantic models has a long history. Suffice it to say that the well-known Markov chains were first introduced as one of such models. The representation of biological data as text and, consequently, applications of text-analysis models in the field of comparative genomics are substantially newer; nevertheless the methods are well developed. In this book, we try to juxtapose linguistic and bioinformatics models of text analysis. So, it can be read, in a sense, “in two directions” – the book is written so as to appeal to the bioinformatician, who may be interested in finding techniques that had initially appeared in the natural language analysis, and to computational linguist, who may be surprised to discover familiar methods used in bioinformatics. In the presentation of the material, the authors, nevertheless, give preference their professional field - bioinformatics. Therefore, even a specialist in bioinformatics can find something new himself in this book. For example, this book includes a review of the main data mining models generating the text spectra. The chapters of the book assume neither advanced mathematical skills nor beginner knowledge of molecular biology. Relevant biological concepts are introduced in the beginning of the book. Several computer science issues relevant to the topics of the book are reviewed in the three appendices: clustering, sequence complexity, and DNA curvature modeling.
Content:
Front Matter....Pages -
Biological Background....Pages 1-16
Biological Classification....Pages 17-22
Mathematical Models for the Analysis of Natural-Language Documents....Pages 23-42
DNA Texts....Pages 43-60
N-Gram Spectra of the DNA Text....Pages 61-85
Application of Compositional Spectra to DNA Sequences....Pages 87-112
Marker-Function Profile-Based Clustering....Pages 113-145
Genome as a Bag of Genes – The Whole-Genome Phylogenetics....Pages 147-160
Back Matter....Pages -
....