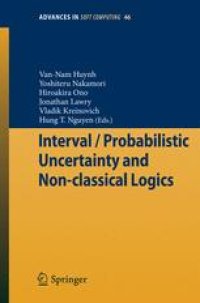
Ebook: Interval / Probabilistic Uncertainty and Non-Classical Logics
- Tags: Appl.Mathematics/Computational Methods of Engineering, Artificial Intelligence (incl. Robotics), Applications of Mathematics, Statistics for Engineering Physics Computer Science Chemistry & Geosciences
- Series: Advances in Soft Computing 46
- Year: 2008
- Publisher: Springer-Verlag Berlin Heidelberg
- Edition: 1
- Language: English
- pdf
Most successful applications of modern science and engineering, from discovering the human genome to predicting weather to controlling space missions, involve processing large amounts of data and large knowledge bases. The ability of computers to perform fast data and knowledge processing is based on the hardware support for super-fast elementary computer operations, such as performing arithmetic operations with (exactly known) numbers and performing logical operations with binary ("true"-"false") logical values. In practice, measurements are never 100% accurate. It is therefore necessary to find out how this input inaccuracy (uncertainty) affects the results of data processing. Sometimes, we know the corresponding probability distribution; sometimes, we only know the upper bounds on the measurement error -- which leads to interval bounds on the (unknown) actual value. Also, experts are usually not 100% certain about the statements included in the knowledge bases. A natural way to describe this uncertainty is to use non-classical logics (probabilistic, fuzzy, etc.).
This book contains proceedings of the first international workshop that brought together researchers working on interval and probabilistic uncertainty and on non-classical logics. We hope that this workshop will lead to a boost in the much-needed collaboration between the uncertainty analysis and non-classical logic communities, and thus, to better processing of uncertainty.
Most successful applications of modern science and engineering, from discovering the human genome to predicting weather to controlling space missions, involve processing large amounts of data and large knowledge bases. The ability of computers to perform fast data and knowledge processing is based on the hardware support for super-fast elementary computer operations, such as performing arithmetic operations with (exactly known) numbers and performing logical operations with binary ("true"-"false") logical values. In practice, measurements are never 100% accurate. It is therefore necessary to find out how this input inaccuracy (uncertainty) affects the results of data processing. Sometimes, we know the corresponding probability distribution; sometimes, we only know the upper bounds on the measurement error -- which leads to interval bounds on the (unknown) actual value. Also, experts are usually not 100% certain about the statements included in the knowledge bases. A natural way to describe this uncertainty is to use non-classical logics (probabilistic, fuzzy, etc.).
This book contains proceedings of the first international workshop that brought together researchers working on interval and probabilistic uncertainty and on non-classical logics. We hope that this workshop will lead to a boost in the much-needed collaboration between the uncertainty analysis and non-classical logic communities, and thus, to better processing of uncertainty.
Most successful applications of modern science and engineering, from discovering the human genome to predicting weather to controlling space missions, involve processing large amounts of data and large knowledge bases. The ability of computers to perform fast data and knowledge processing is based on the hardware support for super-fast elementary computer operations, such as performing arithmetic operations with (exactly known) numbers and performing logical operations with binary ("true"-"false") logical values. In practice, measurements are never 100% accurate. It is therefore necessary to find out how this input inaccuracy (uncertainty) affects the results of data processing. Sometimes, we know the corresponding probability distribution; sometimes, we only know the upper bounds on the measurement error -- which leads to interval bounds on the (unknown) actual value. Also, experts are usually not 100% certain about the statements included in the knowledge bases. A natural way to describe this uncertainty is to use non-classical logics (probabilistic, fuzzy, etc.).
This book contains proceedings of the first international workshop that brought together researchers working on interval and probabilistic uncertainty and on non-classical logics. We hope that this workshop will lead to a boost in the much-needed collaboration between the uncertainty analysis and non-classical logic communities, and thus, to better processing of uncertainty.
Content:
Front Matter....Pages -
Front Matter....Pages 1-1
An Algebraic Approach to Substructural Logics – An Overview....Pages 3-4
On Modeling of Uncertainty Measures and Observed Processes....Pages 5-15
Front Matter....Pages 17-17
Fast Algorithms for Computing Statistics under Interval Uncertainty: An Overview....Pages 19-31
Trade-Off between Sample Size and Accuracy: Case of Static Measurements under Interval Uncertainty....Pages 32-44
Trade-Off between Sample Size and Accuracy: Case of Dynamic Measurements under Interval Uncertainty....Pages 45-56
Estimating Quality of Support Vector Machines Learning under Probabilistic and Interval Uncertainty: Algorithms and Computational Complexity....Pages 57-69
Imprecise Probability as an Approach to Improved Dependability in High-Level Information Fusion....Pages 70-84
Front Matter....Pages 85-85
Label Semantics as a Framework for Granular Modelling....Pages 87-102
Approximating Reasoning for Fuzzy-Based Information Retrieval....Pages 103-114
Probabilistic Constraints for Inverse Problems....Pages 115-128
The Evidential Reasoning Approach for Multi-attribute Decision Analysis under Both Fuzzy and Interval Uncertainty....Pages 129-140
Modelling and Computing with Imprecise and Uncertain Properties in Object Bases....Pages 141-159
Front Matter....Pages 161-161
Several Reducts in Dominance-Based Rough Set Approach....Pages 163-175
Topologies of Approximation Spaces of Rough Set Theory....Pages 176-186
Uncertainty Reasoning in Rough Knowledge Discovery....Pages 187-200
Semantics of the Relative Belief of Singletons....Pages 201-213
A Lattice-Theoretic Interpretation of Independence of Frames....Pages 214-227
Front Matter....Pages 229-229
Completions of Ordered Algebraic Structures: A Survey....Pages 231-244
The Algebra of Truth Values of Type-2 Fuzzy Sets: A Survey....Pages 245-255
Some Properties of Logic Functions over Multi-interval Truth Values....Pages 256-267
Front Matter....Pages 229-229
Possible Semantics for a Common Framework of Probabilistic Logics....Pages 268-279
A Unified Formulation of Deduction, Induction and Abduction Using Granularity Based on VPRS Models and Measure-Based Semantics for Modal Logics....Pages 280-290
Information from Inconsistent Knowledge: A Probability Logic Approach....Pages 291-307
Front Matter....Pages 309-309
Personalized Recommendation for Traditional Crafts Using Fuzzy Correspondence Analysis with Kansei Data and OWA Operator....Pages 311-325
A Probability-Based Approach to Consumer Oriented Evaluation of Traditional Craft Items Using Kansai Data....Pages 326-340
Using Interval Function Approximation to Estimate Uncertainty....Pages 341-352
Interval Forecasting of Crude Oil Price....Pages 353-363
Automatic Classification for Decision Making of the Severeness of the Acute Radiation Syndrome....Pages 364-371
Back Matter....Pages -
Most successful applications of modern science and engineering, from discovering the human genome to predicting weather to controlling space missions, involve processing large amounts of data and large knowledge bases. The ability of computers to perform fast data and knowledge processing is based on the hardware support for super-fast elementary computer operations, such as performing arithmetic operations with (exactly known) numbers and performing logical operations with binary ("true"-"false") logical values. In practice, measurements are never 100% accurate. It is therefore necessary to find out how this input inaccuracy (uncertainty) affects the results of data processing. Sometimes, we know the corresponding probability distribution; sometimes, we only know the upper bounds on the measurement error -- which leads to interval bounds on the (unknown) actual value. Also, experts are usually not 100% certain about the statements included in the knowledge bases. A natural way to describe this uncertainty is to use non-classical logics (probabilistic, fuzzy, etc.).
This book contains proceedings of the first international workshop that brought together researchers working on interval and probabilistic uncertainty and on non-classical logics. We hope that this workshop will lead to a boost in the much-needed collaboration between the uncertainty analysis and non-classical logic communities, and thus, to better processing of uncertainty.
Content:
Front Matter....Pages -
Front Matter....Pages 1-1
An Algebraic Approach to Substructural Logics – An Overview....Pages 3-4
On Modeling of Uncertainty Measures and Observed Processes....Pages 5-15
Front Matter....Pages 17-17
Fast Algorithms for Computing Statistics under Interval Uncertainty: An Overview....Pages 19-31
Trade-Off between Sample Size and Accuracy: Case of Static Measurements under Interval Uncertainty....Pages 32-44
Trade-Off between Sample Size and Accuracy: Case of Dynamic Measurements under Interval Uncertainty....Pages 45-56
Estimating Quality of Support Vector Machines Learning under Probabilistic and Interval Uncertainty: Algorithms and Computational Complexity....Pages 57-69
Imprecise Probability as an Approach to Improved Dependability in High-Level Information Fusion....Pages 70-84
Front Matter....Pages 85-85
Label Semantics as a Framework for Granular Modelling....Pages 87-102
Approximating Reasoning for Fuzzy-Based Information Retrieval....Pages 103-114
Probabilistic Constraints for Inverse Problems....Pages 115-128
The Evidential Reasoning Approach for Multi-attribute Decision Analysis under Both Fuzzy and Interval Uncertainty....Pages 129-140
Modelling and Computing with Imprecise and Uncertain Properties in Object Bases....Pages 141-159
Front Matter....Pages 161-161
Several Reducts in Dominance-Based Rough Set Approach....Pages 163-175
Topologies of Approximation Spaces of Rough Set Theory....Pages 176-186
Uncertainty Reasoning in Rough Knowledge Discovery....Pages 187-200
Semantics of the Relative Belief of Singletons....Pages 201-213
A Lattice-Theoretic Interpretation of Independence of Frames....Pages 214-227
Front Matter....Pages 229-229
Completions of Ordered Algebraic Structures: A Survey....Pages 231-244
The Algebra of Truth Values of Type-2 Fuzzy Sets: A Survey....Pages 245-255
Some Properties of Logic Functions over Multi-interval Truth Values....Pages 256-267
Front Matter....Pages 229-229
Possible Semantics for a Common Framework of Probabilistic Logics....Pages 268-279
A Unified Formulation of Deduction, Induction and Abduction Using Granularity Based on VPRS Models and Measure-Based Semantics for Modal Logics....Pages 280-290
Information from Inconsistent Knowledge: A Probability Logic Approach....Pages 291-307
Front Matter....Pages 309-309
Personalized Recommendation for Traditional Crafts Using Fuzzy Correspondence Analysis with Kansei Data and OWA Operator....Pages 311-325
A Probability-Based Approach to Consumer Oriented Evaluation of Traditional Craft Items Using Kansai Data....Pages 326-340
Using Interval Function Approximation to Estimate Uncertainty....Pages 341-352
Interval Forecasting of Crude Oil Price....Pages 353-363
Automatic Classification for Decision Making of the Severeness of the Acute Radiation Syndrome....Pages 364-371
Back Matter....Pages -
....