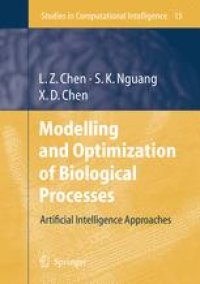
Ebook: Modelling and Optimization of Biotechnological Processes: Artificial Intelligence Approaches
- Tags: Appl.Mathematics/Computational Methods of Engineering, Artificial Intelligence (incl. Robotics), Biomedical Engineering, Bioinformatics
- Series: Studies in Computational Intelligence 15
- Year: 2006
- Publisher: Springer-Verlag Berlin Heidelberg
- Edition: 1
- Language: English
- pdf
This book presents logical approaches to monitoring, modelling and optimization of fed-batch fermentation processes based on artificial intelligence methods, in particular, neural networks and genetic algorithms. Both computer simulation and experimental validation are demonstrated in this book. The approaches proposed in this book can be readily adopted for different processes and control schemes to achieve maximum productivity with minimum development and production costs. These approaches can eliminate the difficulties of having to specify completely the structures and parameters of highly nonlinear bioprocess models.
The book begins with a historical introduction to the field of bioprocess control based on artificial intelligence approaches, followed by two chapters covering the optimization of fed-batch culture using genetic algorithms. Online biomass soft-sensors are constructed in Chapter 4 using recurrent neural networks. The bioprocess is then modelled in Chapter 5 by cascading two soft-sensor neural networks. Optimization and validation of the final product are detailed in Chapters 6 and 7. The general conclusions are drawn in Chapter 8.
This book presents logical approaches to monitoring, modelling and optimization of fed-batch fermentation processes based on artificial intelligence methods, in particular, neural networks and genetic algorithms. Both computer simulation and experimental validation are demonstrated in this book. The approaches proposed in this book can be readily adopted for different processes and control schemes to achieve maximum productivity with minimum development and production costs. These approaches can eliminate the difficulties of having to specify completely the structures and parameters of highly nonlinear bioprocess models.
The book begins with a historical introduction to the field of bioprocess control based on artificial intelligence approaches, followed by two chapters covering the optimization of fed-batch culture using genetic algorithms. Online biomass soft-sensors are constructed in Chapter 4 using recurrent neural networks. The bioprocess is then modelled in Chapter 5 by cascading two soft-sensor neural networks. Optimization and validation of the final product are detailed in Chapters 6 and 7. The general conclusions are drawn in Chapter 8.
This book presents logical approaches to monitoring, modelling and optimization of fed-batch fermentation processes based on artificial intelligence methods, in particular, neural networks and genetic algorithms. Both computer simulation and experimental validation are demonstrated in this book. The approaches proposed in this book can be readily adopted for different processes and control schemes to achieve maximum productivity with minimum development and production costs. These approaches can eliminate the difficulties of having to specify completely the structures and parameters of highly nonlinear bioprocess models.
The book begins with a historical introduction to the field of bioprocess control based on artificial intelligence approaches, followed by two chapters covering the optimization of fed-batch culture using genetic algorithms. Online biomass soft-sensors are constructed in Chapter 4 using recurrent neural networks. The bioprocess is then modelled in Chapter 5 by cascading two soft-sensor neural networks. Optimization and validation of the final product are detailed in Chapters 6 and 7. The general conclusions are drawn in Chapter 8.
Content:
Front Matter....Pages I-VIII
Introduction....Pages 1-16
Optimization of Fed-batch Culture of Hybridoma Cells using Genetic Algorithms....Pages 17-27
On-line Identification and Optimization of Feed Rate Profiles for Fed-batch Culture of Hybridoma Cells....Pages 29-40
On-line Softsensor Development for Biomass Measurements using Dynamic Neural Networks....Pages 41-56
Optimization of Fed-batch Fermentation Processes using Genetic Algorithms based on Cascade Dynamic Neural Network Models....Pages 57-70
Experimental Validation of Cascade Recurrent Neural Network Models....Pages 71-89
Designing and Implementing Optimal Control of Fed-batch Fermentation Processes....Pages 91-108
Conclusions....Pages 109-110
Back Matter....Pages 111-123
This book presents logical approaches to monitoring, modelling and optimization of fed-batch fermentation processes based on artificial intelligence methods, in particular, neural networks and genetic algorithms. Both computer simulation and experimental validation are demonstrated in this book. The approaches proposed in this book can be readily adopted for different processes and control schemes to achieve maximum productivity with minimum development and production costs. These approaches can eliminate the difficulties of having to specify completely the structures and parameters of highly nonlinear bioprocess models.
The book begins with a historical introduction to the field of bioprocess control based on artificial intelligence approaches, followed by two chapters covering the optimization of fed-batch culture using genetic algorithms. Online biomass soft-sensors are constructed in Chapter 4 using recurrent neural networks. The bioprocess is then modelled in Chapter 5 by cascading two soft-sensor neural networks. Optimization and validation of the final product are detailed in Chapters 6 and 7. The general conclusions are drawn in Chapter 8.
Content:
Front Matter....Pages I-VIII
Introduction....Pages 1-16
Optimization of Fed-batch Culture of Hybridoma Cells using Genetic Algorithms....Pages 17-27
On-line Identification and Optimization of Feed Rate Profiles for Fed-batch Culture of Hybridoma Cells....Pages 29-40
On-line Softsensor Development for Biomass Measurements using Dynamic Neural Networks....Pages 41-56
Optimization of Fed-batch Fermentation Processes using Genetic Algorithms based on Cascade Dynamic Neural Network Models....Pages 57-70
Experimental Validation of Cascade Recurrent Neural Network Models....Pages 71-89
Designing and Implementing Optimal Control of Fed-batch Fermentation Processes....Pages 91-108
Conclusions....Pages 109-110
Back Matter....Pages 111-123
....