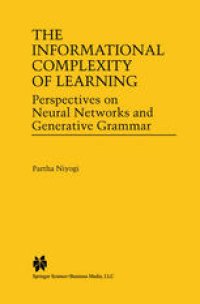
Ebook: The Informational Complexity of Learning: Perspectives on Neural Networks and Generative Grammar
Author: Partha Niyogi (auth.)
- Tags: Artificial Intelligence (incl. Robotics), Linguistics (general), Theory of Computation, Signal Image and Speech Processing
- Year: 1998
- Publisher: Springer US
- Edition: 1
- Language: English
- pdf
Among other topics, The Informational Complexity of Learning:Perspectives on Neural Networks and Generative Grammar brings together two important but very different learning problems within the same analytical framework. The first concerns the problem of learning functional mappings using neural networks, followed by learning natural language grammars in the principles and parameters tradition of Chomsky.
These two learning problems are seemingly very different. Neural networks are real-valued, infinite-dimensional, continuous mappings. On the other hand, grammars are boolean-valued, finite-dimensional, discrete (symbolic) mappings. Furthermore the research communities that work in the two areas almost never overlap.
The book's objective is to bridge this gap. It uses the formal techniques developed in statistical learning theory and theoretical computer science over the last decade to analyze both kinds of learning problems. By asking the same question - how much information does it take to learn? - of both problems, it highlights their similarities and differences. Specific results include model selection in neural networks, active learning, language learning and evolutionary models of language change.
The Informational Complexity of Learning: Perspectives on NeuralNetworks and Generative Grammar is a very interdisciplinary work. Anyone interested in the interaction of computer science and cognitive science should enjoy the book. Researchers in artificial intelligence, neural networks, linguistics, theoretical computer science, and statistics will find it particularly relevant.
Among other topics, The Informational Complexity of Learning:Perspectives on Neural Networks and Generative Grammar brings together two important but very different learning problems within the same analytical framework. The first concerns the problem of learning functional mappings using neural networks, followed by learning natural language grammars in the principles and parameters tradition of Chomsky.
These two learning problems are seemingly very different. Neural networks are real-valued, infinite-dimensional, continuous mappings. On the other hand, grammars are boolean-valued, finite-dimensional, discrete (symbolic) mappings. Furthermore the research communities that work in the two areas almost never overlap.
The book's objective is to bridge this gap. It uses the formal techniques developed in statistical learning theory and theoretical computer science over the last decade to analyze both kinds of learning problems. By asking the same question - how much information does it take to learn? - of both problems, it highlights their similarities and differences. Specific results include model selection in neural networks, active learning, language learning and evolutionary models of language change.
The Informational Complexity of Learning: Perspectives on NeuralNetworks and Generative Grammar is a very interdisciplinary work. Anyone interested in the interaction of computer science and cognitive science should enjoy the book. Researchers in artificial intelligence, neural networks, linguistics, theoretical computer science, and statistics will find it particularly relevant.
Among other topics, The Informational Complexity of Learning:Perspectives on Neural Networks and Generative Grammar brings together two important but very different learning problems within the same analytical framework. The first concerns the problem of learning functional mappings using neural networks, followed by learning natural language grammars in the principles and parameters tradition of Chomsky.
These two learning problems are seemingly very different. Neural networks are real-valued, infinite-dimensional, continuous mappings. On the other hand, grammars are boolean-valued, finite-dimensional, discrete (symbolic) mappings. Furthermore the research communities that work in the two areas almost never overlap.
The book's objective is to bridge this gap. It uses the formal techniques developed in statistical learning theory and theoretical computer science over the last decade to analyze both kinds of learning problems. By asking the same question - how much information does it take to learn? - of both problems, it highlights their similarities and differences. Specific results include model selection in neural networks, active learning, language learning and evolutionary models of language change.
The Informational Complexity of Learning: Perspectives on NeuralNetworks and Generative Grammar is a very interdisciplinary work. Anyone interested in the interaction of computer science and cognitive science should enjoy the book. Researchers in artificial intelligence, neural networks, linguistics, theoretical computer science, and statistics will find it particularly relevant.
Content:
Front Matter....Pages i-xxiii
Introduction....Pages 1-19
On the Relationship between Hyothesis Complexity, Sample Complexity and Generalization Error for Neural Networks....Pages 21-73
Investigating the Sample Complexity of Active Learning Schemes....Pages 75-123
Language Learning Problems in the Principles and Parameters Framework....Pages 125-171
The Logical Problem of Language Change....Pages 173-205
Conclusions....Pages 207-212
Back Matter....Pages 213-224
Among other topics, The Informational Complexity of Learning:Perspectives on Neural Networks and Generative Grammar brings together two important but very different learning problems within the same analytical framework. The first concerns the problem of learning functional mappings using neural networks, followed by learning natural language grammars in the principles and parameters tradition of Chomsky.
These two learning problems are seemingly very different. Neural networks are real-valued, infinite-dimensional, continuous mappings. On the other hand, grammars are boolean-valued, finite-dimensional, discrete (symbolic) mappings. Furthermore the research communities that work in the two areas almost never overlap.
The book's objective is to bridge this gap. It uses the formal techniques developed in statistical learning theory and theoretical computer science over the last decade to analyze both kinds of learning problems. By asking the same question - how much information does it take to learn? - of both problems, it highlights their similarities and differences. Specific results include model selection in neural networks, active learning, language learning and evolutionary models of language change.
The Informational Complexity of Learning: Perspectives on NeuralNetworks and Generative Grammar is a very interdisciplinary work. Anyone interested in the interaction of computer science and cognitive science should enjoy the book. Researchers in artificial intelligence, neural networks, linguistics, theoretical computer science, and statistics will find it particularly relevant.
Content:
Front Matter....Pages i-xxiii
Introduction....Pages 1-19
On the Relationship between Hyothesis Complexity, Sample Complexity and Generalization Error for Neural Networks....Pages 21-73
Investigating the Sample Complexity of Active Learning Schemes....Pages 75-123
Language Learning Problems in the Principles and Parameters Framework....Pages 125-171
The Logical Problem of Language Change....Pages 173-205
Conclusions....Pages 207-212
Back Matter....Pages 213-224
....