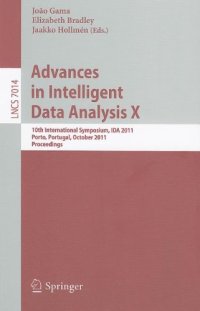
Ebook: Advances in Intelligent Data Analysis X: 10th International Symposium, IDA 2011, Porto, Portugal, October 29-31, 2011. Proceedings
- Tags: Database Management, Information Systems Applications (incl. Internet), Artificial Intelligence (incl. Robotics), Information Storage and Retrieval, Algorithm Analysis and Problem Complexity, Data Mining and Knowledge Discovery
- Series: Lecture Notes in Computer Science 7014
- Year: 2011
- Publisher: Springer-Verlag Berlin Heidelberg
- Edition: 1
- Language: English
- pdf
This book constitutes the refereed proceedings of the 10th International Conference on Intelligent Data Analysis, IDA 2011, held in Porto, Portugal, in October 2011. The 19 revised full papers and 16 revised poster papers resented together with 3 invited papers were carefully reviewed and selected from 73 submissions. All current aspects of intelligent data analysis are addressed, particularly intelligent support for modeling and analyzing complex, dynamical systems. The papers offer intelligent support for understanding evolving scientific and social systems including data collection and acquisition, such as crowd sourcing; data cleaning, semantics and markup; searching for data and assembling datasets from multiple sources; data processing, including workflows, mixed-initiative data analysis, and planning; data and information fusion; incremental, mixed-initiative model development, testing and revision; and visualization and dissemination of results; etc.
This book constitutes the refereed proceedings of the 10th International Conference on Intelligent Data Analysis, IDA 2011, held in Porto, Portugal, in October 2011. The 19 revised full papers and 16 revised poster papers resented together with 3 invited papers were carefully reviewed and selected from 73 submissions. All current aspects of intelligent data analysis are addressed, particularly intelligent support for modeling and analyzing complex, dynamical systems. The papers offer intelligent support for understanding evolving scientific and social systems including data collection and acquisition, such as crowd sourcing; data cleaning, semantics and markup; searching for data and assembling datasets from multiple sources; data processing, including workflows, mixed-initiative data analysis, and planning; data and information fusion; incremental, mixed-initiative model development, testing and revision; and visualization and dissemination of results; etc.