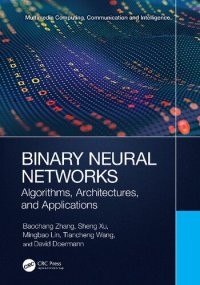
Ebook: Binary Neural Networks: Algorithms, Architectures, and Application
- Genre: Computers // Algorithms and Data Structures: Pattern Recognition
- Year: 2023
- Publisher: CRC Press
- Language: English
- pdf
Deep Learning has achieved impressive results in image classification, computer vision, and natural language processing. To achieve better performance, deeper and wider networks have been designed, which increase the demand for computational resources. The number of floatingpoint operations (FLOPs) has increased dramatically with larger networks, and this has become an obstacle for convolutional neural networks (CNNs) being developed for mobile and embedded devices. In this context, Binary Neural Networks: Algorithms, Architectures, and Applications will focus on CNN compression and acceleration, which are important for the research community. We will describe numerous methods, including parameter quantization, network pruning, low-rank decomposition, and knowledge distillation.
More recently, to reduce the burden of handcrafted architecture design, neural architecture search (NAS) has been used to automatically build neural networks by searching over a vast architecture space. Our book will also introduce NAS and its superiority and state-of-the-art performance in various applications, such as image classification and object detection. We also describe extensive applications of compressed deep models on image classification, speech recognition, object detection, and tracking. These topics can help researchers better understand the usefulness and the potential of network compression on practical applications. Moreover, interested readers should have basic knowledge of Machine Learning and Deep Learning to better understand the methods described in this book.
Deep Learning has become increasingly important because of its superior performance. Still, it suffers from a large memory footprint and high computational cost, making it difficult to deploy on front-end devices. For example, in unmanned systems, UAVs serve as computing terminals with limited memory and computing resources, making it difficult to perform real-time data processing based on convolutional neural networks (CNNs). To improve storage and computation efficiency, BNNs have shown promise for practical applications. BNNs are neural networks where the weights are binarized. 1-bit CNNs are a highly compressed version of BNNs that binarize both the weights and the activations to decrease the model size and computational cost. These highly compressed models make them suitable for front-end computing. In addition to these two, other quantizing neural networks, such as pruning and sparse neural networks, are widely used in edge computing.
Key Features:
• Reviews recent advances in CNN compression and acceleration
• Elaborates recent advances on binary neural network (BNN) technologies
• Introduces applications of BNN in image classification, speech recognition, object detection, and more
More recently, to reduce the burden of handcrafted architecture design, neural architecture search (NAS) has been used to automatically build neural networks by searching over a vast architecture space. Our book will also introduce NAS and its superiority and state-of-the-art performance in various applications, such as image classification and object detection. We also describe extensive applications of compressed deep models on image classification, speech recognition, object detection, and tracking. These topics can help researchers better understand the usefulness and the potential of network compression on practical applications. Moreover, interested readers should have basic knowledge of Machine Learning and Deep Learning to better understand the methods described in this book.
Deep Learning has become increasingly important because of its superior performance. Still, it suffers from a large memory footprint and high computational cost, making it difficult to deploy on front-end devices. For example, in unmanned systems, UAVs serve as computing terminals with limited memory and computing resources, making it difficult to perform real-time data processing based on convolutional neural networks (CNNs). To improve storage and computation efficiency, BNNs have shown promise for practical applications. BNNs are neural networks where the weights are binarized. 1-bit CNNs are a highly compressed version of BNNs that binarize both the weights and the activations to decrease the model size and computational cost. These highly compressed models make them suitable for front-end computing. In addition to these two, other quantizing neural networks, such as pruning and sparse neural networks, are widely used in edge computing.
Key Features:
• Reviews recent advances in CNN compression and acceleration
• Elaborates recent advances on binary neural network (BNN) technologies
• Introduces applications of BNN in image classification, speech recognition, object detection, and more
Download the book Binary Neural Networks: Algorithms, Architectures, and Application for free or read online
Continue reading on any device:
Last viewed books
Related books
{related-news}
Comments (0)