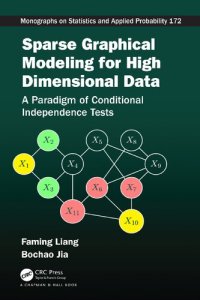
Ebook: Sparse Graphical Modeling for High Dimensional Data: A Paradigm of Conditional Independence Tests
Author: Faming Liang Bochao Jia
- Genre: Science (General) // Science of Science
- Series: Chapman & Hall/CRC Monographs on Statistics and Applied Probability 172
- Year: 2023
- Publisher: CRC Press/Chapman & Hall
- City: Boca Raton
- Language: English
- pdf
This book provides a general framework for learning sparse graphical models with conditional independence tests. It includes complete treatments for Gaussian, Poisson, multinomial, and mixed data; unified treatments for covariate adjustments, data integration, and network comparison; unified treatments for missing data and heterogeneous data; efficient methods for joint estimation of multiple graphical models; effective methods of high-dimensional variable selection; and effective methods of high-dimensional inference. The methods possess an embarrassingly parallel structure in performing conditional independence tests, and the computation can be significantly accelerated by running in parallel on a multi-core computer or a parallel architecture. This book is intended to serve researchers and scientists interested in high-dimensional statistics, and graduate students in broad data science disciplines.
Key Features:
- A general framework for learning sparse graphical models with conditional independence tests
- Complete treatments for different types of data, Gaussian, Poisson, multinomial, and mixed data
- Unified treatments for data integration, network comparison, and covariate adjustment
- Unified treatments for missing data and heterogeneous data
- Efficient methods for joint estimation of multiple graphical models
- Effective methods of high-dimensional variable selection
- Effective methods of high-dimensional inference