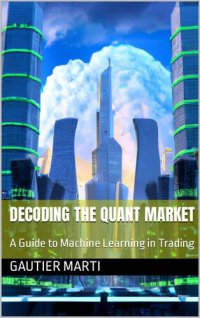
Ebook: Decoding the Quant Market: A Guide to Machine Learning in Trading
Author: Gautier Marti
- Genre: Computers // Algorithms and Data Structures: Pattern Recognition
- Year: 2023
- Publisher: Independently published
- Language: English
- azw3
In the ever-changing world of finance and trading, the search for a competitive edge has been a constant driver of innovation. Over the last few decades, the field of quantitative trading has emerged as a powerful force, pushing the boundaries of what is possible and reshaping the way we approach the market. At the heart of this transformation lies the fusion of cutting-edge technology, data-driven insights, and the unwavering curiosity of the human mind. It is this intersection of disciplines that forms the foundation for ”Decoding the Quant Market: A Guide to Machine Learning in Trading.”
In this book, I aim to share my experiences and insights, offering a comprehensive guide to navigating the world of Machine Learning in quantitative trading. The journey begins with a foundational understanding of the core principles, theories, and algorithms that have shaped the field. From there, we delve into the practical applications of these techniques, exploring real-world examples and case studies that illustrate the power of Machine Learning (ML) in trading.
”Decoding the Quant Market” is designed to be accessible to readers from diverse backgrounds, whether they are seasoned professionals or newcomers to the field of finance and technology. By combining theoretical knowledge with practical insights and examples, this book aims to provide a well-rounded understanding of the complex world of Machine Learning in trading.
Supervised learning is a type of Machine Learning where an algorithm is trained on a labeled dataset, containing both input features and corresponding output targets. The algorithm learns a mapping between the features and targets, which can then be used to make predictions on unseen data. In trading, features can be any relevant market data or derived statistics, such as technical indicators, fundamental data, sentiment analysis, or even macroeconomic indicators. Targets, on the other hand, represent the outcomes we aim to predict, such as future price movements or trade signals. The combination of features and targets in historical data forms the training dataset used to teach the supervised learning algorithm.
Deep Learning (DL) is a subset of Machine Learning that focuses on neural networks with multiple hidden layers, allowing the model to learn complex representations of the input data. Neural networks consist of layers of interconnected neurons, which process input data and transform it into output predictions. The key components of neural networks include layers (input, hidden, and output), neurons, and activation functions. The learning process involves forward propagation, where input data is passed through the network to generate predictions, and backpropagation, where the model’s weights are updated to minimize the prediction error.
Deep Learning can be applied to various trading problems, such as:
• Time series forecasting with Recurrent Neural Networks (RNNs): RNNs are designed to handle sequential data by maintaining an internal state that can capture information from previous time steps. In trading, RNNs can be used to predict future asset prices or volatility based on historical data.
• Convolutional Neural Networks (CNNs) for pattern recognition: CNNs are designed to process grid-like data, such as images or time series, by applying convolutional filters that can detect local patterns. In trading, CNNs can be used to recognize technical patterns, such as chart formations or trends, and generate trade signals accordingly.
• Graph Neural Networks (GNNs) for relational analysis: GNNs are designed to handle graph-structured data, capturing complex relationships between entities. In trading, GNNs can be used to model the relationships between different assets, industries, or macroeconomic factors, allowing traders to identify potential opportunities or risks arising from these connections.
• Transformers for sequence analysis: Transformers are a type of deep learning model that have been highly successful in natural language processing tasks. They are designed to handle sequential data by utilizing self-attention mechanisms, allowing them to capture long-range dependencies in the input data. In trading, transformers can be used for time series forecasting, sentiment analysis, and event detection in financial texts.
These Deep Learning techniques offer powerful solutions for traders to tackle a wide range of trading problems. By leveraging these advanced models, traders can gain deeper insights into the market, uncover hidden patterns, and develop more effective trading strategies.
In this book, I aim to share my experiences and insights, offering a comprehensive guide to navigating the world of Machine Learning in quantitative trading. The journey begins with a foundational understanding of the core principles, theories, and algorithms that have shaped the field. From there, we delve into the practical applications of these techniques, exploring real-world examples and case studies that illustrate the power of Machine Learning (ML) in trading.
”Decoding the Quant Market” is designed to be accessible to readers from diverse backgrounds, whether they are seasoned professionals or newcomers to the field of finance and technology. By combining theoretical knowledge with practical insights and examples, this book aims to provide a well-rounded understanding of the complex world of Machine Learning in trading.
Supervised learning is a type of Machine Learning where an algorithm is trained on a labeled dataset, containing both input features and corresponding output targets. The algorithm learns a mapping between the features and targets, which can then be used to make predictions on unseen data. In trading, features can be any relevant market data or derived statistics, such as technical indicators, fundamental data, sentiment analysis, or even macroeconomic indicators. Targets, on the other hand, represent the outcomes we aim to predict, such as future price movements or trade signals. The combination of features and targets in historical data forms the training dataset used to teach the supervised learning algorithm.
Deep Learning (DL) is a subset of Machine Learning that focuses on neural networks with multiple hidden layers, allowing the model to learn complex representations of the input data. Neural networks consist of layers of interconnected neurons, which process input data and transform it into output predictions. The key components of neural networks include layers (input, hidden, and output), neurons, and activation functions. The learning process involves forward propagation, where input data is passed through the network to generate predictions, and backpropagation, where the model’s weights are updated to minimize the prediction error.
Deep Learning can be applied to various trading problems, such as:
• Time series forecasting with Recurrent Neural Networks (RNNs): RNNs are designed to handle sequential data by maintaining an internal state that can capture information from previous time steps. In trading, RNNs can be used to predict future asset prices or volatility based on historical data.
• Convolutional Neural Networks (CNNs) for pattern recognition: CNNs are designed to process grid-like data, such as images or time series, by applying convolutional filters that can detect local patterns. In trading, CNNs can be used to recognize technical patterns, such as chart formations or trends, and generate trade signals accordingly.
• Graph Neural Networks (GNNs) for relational analysis: GNNs are designed to handle graph-structured data, capturing complex relationships between entities. In trading, GNNs can be used to model the relationships between different assets, industries, or macroeconomic factors, allowing traders to identify potential opportunities or risks arising from these connections.
• Transformers for sequence analysis: Transformers are a type of deep learning model that have been highly successful in natural language processing tasks. They are designed to handle sequential data by utilizing self-attention mechanisms, allowing them to capture long-range dependencies in the input data. In trading, transformers can be used for time series forecasting, sentiment analysis, and event detection in financial texts.
These Deep Learning techniques offer powerful solutions for traders to tackle a wide range of trading problems. By leveraging these advanced models, traders can gain deeper insights into the market, uncover hidden patterns, and develop more effective trading strategies.
Download the book Decoding the Quant Market: A Guide to Machine Learning in Trading for free or read online
Continue reading on any device:
Last viewed books
Related books
{related-news}
Comments (0)