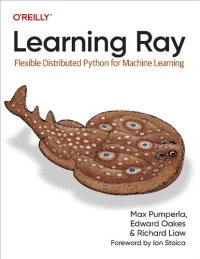
Ebook: Learning Ray: Flexible Distributed Python for Machine Learning (Final Release)
- Genre: Computers // Algorithms and Data Structures: Pattern Recognition
- Year: 2023
- Publisher: O’Reilly Media
- Edition: final
- Language: English
- pdf
Get started with Ray, the open source distributed computing framework that simplifies the process of scaling compute-intensive Python workloads. With this practical book, Python programmers, data engineers, and data scientists will learn how to leverage Ray locally and spin up compute clusters. You'll be able to use Ray to structure and run machine learning programs at scale.
Authors Max Pumperla, Edward Oakes, and Richard Liaw show you how to build machine learning applications with Ray. You'll understand how Ray fits into the current landscape of machine learning tools and discover how Ray continues to integrate ever more tightly with these tools. Distributed computation is hard, but by using Ray you'll find it easy to get started.
Distributed computing is a fascinating topic. Looking back at the early days of computing, I can’t help but be impressed by the fact that so many companies distribute their workloads across clusters of computers. It’s not only impressive because we have figured out efficient ways to do so, but it’s also becoming a necessity. Individual computers keep getting faster and more powerful, and yet our need for large scale computing keeps exceeding what single machines can do.
Ray simplifies distributed computing for non-experts and makes it easy to take Python scripts and scale them across multiple nodes. Ray is great at scaling both data and compute heavy workloads, such as data transformations and model training, and targets machine learning (ML) workloads with the need to scale. The addition of the Ray AI Runtime (AIR) with the release of Ray 2.0 in August 2022 increased the support for complex ML workloads in Ray even further.
Learn how to build your first distributed applications with Ray Core
Conduct hyperparameter optimization with Ray Tune
Use the Ray RLlib library for reinforcement learning
Manage distributed training with the Ray Train library
Use Ray to perform data processing with Ray Datasets
Learn how work with Ray Clusters and serve models with Ray Serve
Build end-to-end machine learning applications with Ray AIR
Who Should Read This Book:
It’s likely that you picked up this book because you’re interested in some aspects of Ray. Maybe you’re a distributed systems engineer who wants to know how Ray’s engine works. You might also be a software developer interested in picking up a new technology. Or you could be a data engineer who wants to evaluate how Ray compares to similar tools. You could also be a machine learning practitioner or data scientist who needs to find ways to scale experiments.
No matter your concrete role, the common denominator to get the most out of this book is to feel comfortable programming in Python. This book’s examples are written in Python, and an intermediate knowledge of the language is a requirement. Explicit is better than implicit, as you know full well as a Pythonista. So, let us be explicit by saying that knowing Python implies to me that you know how to use the command line on your system, how to get help when stuck, and how to set up a programming environment on your own.
If you’ve never worked with distributed systems before, that’s OK. We cover all the basics you need to get started with that in the book. On top of that, you can run most code examples presented here on your laptop. Covering the basics means that we can’t go into too much detail about distributed systems. This book is ultimately focused on application developers using Ray, specifically for Data Science and ML.
For the later chapters of this book, you’ll need some familiarity with ML, but we don’t expect you to have worked in the field. In particular, you should have a basic understanding of the ML paradigm and how it differs from traditional programming. You should also know the basics of using NumPy and Pandas. Also, you should at least feel comfortable reading examples using the popular TensorFlow and PyTorch libraries. It’s enough to follow the flow of the code, on the API level, but you don’t need to know how to write your own models. We cover examples using both dominant deep learning libraries (TensorFlow and PyTorch) to illustrate how you can use Ray for ML workloads, regardless of your preferred framework.
We cover a lot of ground in advanced ML topics, but the main focus is on Ray as a technology and how to use it. The ML examples we discuss might be new to you and could require a second reading, but you can still focus on Ray’s API and how to use it in practice.
Authors Max Pumperla, Edward Oakes, and Richard Liaw show you how to build machine learning applications with Ray. You'll understand how Ray fits into the current landscape of machine learning tools and discover how Ray continues to integrate ever more tightly with these tools. Distributed computation is hard, but by using Ray you'll find it easy to get started.
Distributed computing is a fascinating topic. Looking back at the early days of computing, I can’t help but be impressed by the fact that so many companies distribute their workloads across clusters of computers. It’s not only impressive because we have figured out efficient ways to do so, but it’s also becoming a necessity. Individual computers keep getting faster and more powerful, and yet our need for large scale computing keeps exceeding what single machines can do.
Ray simplifies distributed computing for non-experts and makes it easy to take Python scripts and scale them across multiple nodes. Ray is great at scaling both data and compute heavy workloads, such as data transformations and model training, and targets machine learning (ML) workloads with the need to scale. The addition of the Ray AI Runtime (AIR) with the release of Ray 2.0 in August 2022 increased the support for complex ML workloads in Ray even further.
Learn how to build your first distributed applications with Ray Core
Conduct hyperparameter optimization with Ray Tune
Use the Ray RLlib library for reinforcement learning
Manage distributed training with the Ray Train library
Use Ray to perform data processing with Ray Datasets
Learn how work with Ray Clusters and serve models with Ray Serve
Build end-to-end machine learning applications with Ray AIR
Who Should Read This Book:
It’s likely that you picked up this book because you’re interested in some aspects of Ray. Maybe you’re a distributed systems engineer who wants to know how Ray’s engine works. You might also be a software developer interested in picking up a new technology. Or you could be a data engineer who wants to evaluate how Ray compares to similar tools. You could also be a machine learning practitioner or data scientist who needs to find ways to scale experiments.
No matter your concrete role, the common denominator to get the most out of this book is to feel comfortable programming in Python. This book’s examples are written in Python, and an intermediate knowledge of the language is a requirement. Explicit is better than implicit, as you know full well as a Pythonista. So, let us be explicit by saying that knowing Python implies to me that you know how to use the command line on your system, how to get help when stuck, and how to set up a programming environment on your own.
If you’ve never worked with distributed systems before, that’s OK. We cover all the basics you need to get started with that in the book. On top of that, you can run most code examples presented here on your laptop. Covering the basics means that we can’t go into too much detail about distributed systems. This book is ultimately focused on application developers using Ray, specifically for Data Science and ML.
For the later chapters of this book, you’ll need some familiarity with ML, but we don’t expect you to have worked in the field. In particular, you should have a basic understanding of the ML paradigm and how it differs from traditional programming. You should also know the basics of using NumPy and Pandas. Also, you should at least feel comfortable reading examples using the popular TensorFlow and PyTorch libraries. It’s enough to follow the flow of the code, on the API level, but you don’t need to know how to write your own models. We cover examples using both dominant deep learning libraries (TensorFlow and PyTorch) to illustrate how you can use Ray for ML workloads, regardless of your preferred framework.
We cover a lot of ground in advanced ML topics, but the main focus is on Ray as a technology and how to use it. The ML examples we discuss might be new to you and could require a second reading, but you can still focus on Ray’s API and how to use it in practice.
Download the book Learning Ray: Flexible Distributed Python for Machine Learning (Final Release) for free or read online
Continue reading on any device:
Last viewed books
Related books
{related-news}
Comments (0)