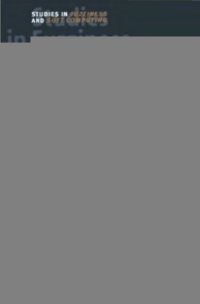
Ebook: Computational Intelligence Systems and Applications. Neuro-Fuzzy and Fuzzy Neural Synergis
Author: Gorzałczany M.B.
- Genre: Computers // Cybernetics: Artificial Intelligence
- Tags: Информатика и вычислительная техника, Искусственный интеллект, Нейронные сети
- Language: English
- pdf
Springer, 2002. — 367.Traditional Artificial Intelligence (AI) systems adopted symbolic processing as their main paradigm. Symbolic AI systems have proved effective in handling problems characterized by exact and complete knowledge representation. Unfortunately, these systems have very little power in dealing with imprecise, uncertain and incomplete data and information which significantly contribute to the description of many real-world problems, both physical systems and processes as well as mechanisms of decision making. Moreover, there are many situations where the expert domain knowledge (the basis for many symbolic AI systems) is not sufficient for the design of intelligent systems, due to incompleteness of the existing knowledge, problems caused by different biases of human experts, difficulties in forming rules, etc.
In general, problem knowledge for solving a given problem can consist of an explicit knowledge (e.g., heuristic rules provided by a domain expert) and an implicit, hidden knowledge "buried" in past-experience numerical data. A study of huge amounts of these data (collected in databases) and the synthesizing of the knowledge "encoded" in them (also referred to as knowledge discovery in data or data mining), can significantly improve the performance of the intelligent systems designed. Since traditional, symbolic AI systems are not able to make effective use of this kind of data, new methods and algorithms for the extraction of knowledge from data, knowledge representation and reasoning have been emerging in the last several years. They can be treated either as complementary techniques with regard to traditional AI systems or as a kind of modern extension and generalization of them. Computational Intelligence (CI) systems - based on various synergistic links between artificial neural networks, methods of granular information processing (in particular, fuzzy sets and fuzzy logic), and methods of evolutionary computations (in particular, genetic algorithms) - are the most representative class of these methodologies.
During the last couple of decades there has been growing interest in algorithms, which rely on analogies to natural processes and "humanlike" problem-solving. All three main constituents of CI systems belong to this group. The theory of fuzzy sets and fuzzy logic was developed as a means for representing, manipulating, and utilizing uncertain information and to provide a framework for handling uncertainties and imprecision in realworld applications. This theory provides inference mechanisms that enable approximate reasoning and model human reasoning capabilities to be applied to knowledge-based intelligent systems. Artificial neural networks are biologically-inspired, massively-parallel, distributed information processing systems. They are characterized by a computational power, fault tolerance, as well as learning and generalizing capabilities. Genetic algorithms are a global-search paradigm based on principles imitating mechanisms of genetics, natural selection, evolution and heredity, including the evolutionary principle of survival of the fittest and extinction of the worst adapted individuals.
Synergistic combination of all three methodologies has a very sound rational basis because they all approach the problem of designing intelligent systems from quite different but complementary angles. Thus, their combination within one system significantly reduces their shortcomings and amplifies their merits. An integrated CI system has the advantages of neural systems (learning, generalization and adaptation abilities, processing huge amounts of numerical data from databases, and a connectionist structure with high fault tolerance and distributed representation properties), fuzzy systems (structural framework with easily -interpretable rule-based knowledge and high-level fuzzy reasoning) and genetic algorithms (parameter and structure optimization of the system).
This research monograph presents new concepts and implementations of CI systems and a broad comparative analysis with several of the existing, best-known neuro-fuzzy systems as well as with systems representing other knowledge-discovery techniques such as rough sets, decision trees, regression trees, probabilistic rule induction, etc. This presentation is preceded by a discussion of the main directions of synthesizing fuzzy sets, artificial neural networks and genetic algorithms in the framework of designing CI systems. In order to keep the book self-contained, introductions to the basic concepts of fuzzy systems, artificial neural networks and genetic algorithms are given. This book is intended for researchers and practitioners in AI/CI fields and for students of computer science or neighbouring areas.Introduction
Elements of the theory of fuzzy sets
Essentials of artificial neural networks
Brief introduction to genetic algorithms
Main directions of combining artificial neural networks, fuzzy sets and evolutionary computations in designing computational intelligence systems
Neuro-fuzzy(-genetic) system for synthesizing rule-based knowledge from data
Rule-based neuro-fuzzy modelling of dynamic systems and designing of controllers
Neuro-fuzzy(-genetic) rule-based classifier designed from data for intelligent decision support
Fuzzy neural network for system modelling and control
Fuzzy neural classifier
In general, problem knowledge for solving a given problem can consist of an explicit knowledge (e.g., heuristic rules provided by a domain expert) and an implicit, hidden knowledge "buried" in past-experience numerical data. A study of huge amounts of these data (collected in databases) and the synthesizing of the knowledge "encoded" in them (also referred to as knowledge discovery in data or data mining), can significantly improve the performance of the intelligent systems designed. Since traditional, symbolic AI systems are not able to make effective use of this kind of data, new methods and algorithms for the extraction of knowledge from data, knowledge representation and reasoning have been emerging in the last several years. They can be treated either as complementary techniques with regard to traditional AI systems or as a kind of modern extension and generalization of them. Computational Intelligence (CI) systems - based on various synergistic links between artificial neural networks, methods of granular information processing (in particular, fuzzy sets and fuzzy logic), and methods of evolutionary computations (in particular, genetic algorithms) - are the most representative class of these methodologies.
During the last couple of decades there has been growing interest in algorithms, which rely on analogies to natural processes and "humanlike" problem-solving. All three main constituents of CI systems belong to this group. The theory of fuzzy sets and fuzzy logic was developed as a means for representing, manipulating, and utilizing uncertain information and to provide a framework for handling uncertainties and imprecision in realworld applications. This theory provides inference mechanisms that enable approximate reasoning and model human reasoning capabilities to be applied to knowledge-based intelligent systems. Artificial neural networks are biologically-inspired, massively-parallel, distributed information processing systems. They are characterized by a computational power, fault tolerance, as well as learning and generalizing capabilities. Genetic algorithms are a global-search paradigm based on principles imitating mechanisms of genetics, natural selection, evolution and heredity, including the evolutionary principle of survival of the fittest and extinction of the worst adapted individuals.
Synergistic combination of all three methodologies has a very sound rational basis because they all approach the problem of designing intelligent systems from quite different but complementary angles. Thus, their combination within one system significantly reduces their shortcomings and amplifies their merits. An integrated CI system has the advantages of neural systems (learning, generalization and adaptation abilities, processing huge amounts of numerical data from databases, and a connectionist structure with high fault tolerance and distributed representation properties), fuzzy systems (structural framework with easily -interpretable rule-based knowledge and high-level fuzzy reasoning) and genetic algorithms (parameter and structure optimization of the system).
This research monograph presents new concepts and implementations of CI systems and a broad comparative analysis with several of the existing, best-known neuro-fuzzy systems as well as with systems representing other knowledge-discovery techniques such as rough sets, decision trees, regression trees, probabilistic rule induction, etc. This presentation is preceded by a discussion of the main directions of synthesizing fuzzy sets, artificial neural networks and genetic algorithms in the framework of designing CI systems. In order to keep the book self-contained, introductions to the basic concepts of fuzzy systems, artificial neural networks and genetic algorithms are given. This book is intended for researchers and practitioners in AI/CI fields and for students of computer science or neighbouring areas.Introduction
Elements of the theory of fuzzy sets
Essentials of artificial neural networks
Brief introduction to genetic algorithms
Main directions of combining artificial neural networks, fuzzy sets and evolutionary computations in designing computational intelligence systems
Neuro-fuzzy(-genetic) system for synthesizing rule-based knowledge from data
Rule-based neuro-fuzzy modelling of dynamic systems and designing of controllers
Neuro-fuzzy(-genetic) rule-based classifier designed from data for intelligent decision support
Fuzzy neural network for system modelling and control
Fuzzy neural classifier
Download the book Computational Intelligence Systems and Applications. Neuro-Fuzzy and Fuzzy Neural Synergis for free or read online
Continue reading on any device:
Last viewed books
Related books
{related-news}
Comments (0)