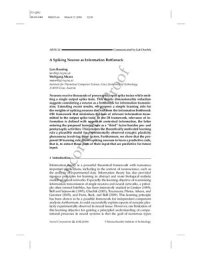
Ebook: A Spiking Neuron as Information Bottleneck
Author: Buesing L. Maass W.
- Genre: Computers // Cybernetics: Artificial Intelligence
- Tags: Информатика и вычислительная техника, Искусственный интеллект, Нейронные сети
- Language: English
- pdf
Neural Computation 22, 1–32 (2010).Neurons receive thousands of presynaptic input spike trains while emitting a single output spike train. This drastic dimensionality reduction suggests considering a neuron as a bottleneck for information transmission.
Extending recent results, we propose a simple learning rule for the weights of spiking neurons derived from the information bottleneck (IB) framework that minimizes the loss of relevant information transmitted in the output spike train. In the IB framework, relevance of information is defined with respect to contextual information, the latter entering the proposed learning rule as a third factor besides pre- and postsynaptic activities. This renders the theoretically motivated learning rule a plausible model for experimentally observed synaptic plasticity phenomena involving three factors. Furthermore, we show that the proposed IB learning rule allows spiking neurons to learn a predictive code, that is, to extract those parts of their input that are predictive for future input.
Information theory is a powerful theoretical framework with numerous important applications, including in the context of neuroscience, such as the analysis of experimental data. Information theory has also provided rigorous principles for learning in abstract and more biological realistic models of neural networks. Especially the learning objective ofmaximizing information transmission of single neurons and neural networks, a principle often termed InfoMax, has been intensively studied in Linsker (1989), Bell and Sejnowski (1995), Chechik (2003), Toyoizumi, Pfister, Aihara, and Gerstner (2005), and Parra, Beck, and Bell (2009). This learning principle has been shown to be a possible framework for independent component analysis; furthermore, it could successfully explain aspects of synaptic plasticity experimentally observed in neural tissue. However, one limitation of this learning objective for gaining a principled understanding of computational processes in neural systems is that the goal of numerous types of computations is not a maximization of information transmission (e.g., from sensory input neurons to areas in the brain where decision are made).
Rather, a characteristic feature of generic computations (e.g., clustering and classification of data, or sorting a list of elements according to some relation) is that they remove some of the information contained in the input. Similarly, generic learning processes require the removal of some of the information originally available in order to achieve generalization capability.
Tishby, Pereira, and Bialek (1999) created a new information-theoretic framework, the information bottleneck (IB) framework, which focuses on transmitting the maximal amount of relevant information. This approach takes a step toward making computational and learning processes more amenable to information-theoretic analysis. We examine in this article whether the IB framework can foster an understanding of organizational principles behind experimentally verified synaptic plasticity mechanisms that involve a third factor" (Sjostrom & Hausser, 2006; Hee et al., 2007).
These are plasticity effects where the amplitude of the synaptic weight change depends not only on the firing activity of the pre- and postsynaptic neuron, but also on a third signal that is transmitted, for example, in the form of neuromodulators or synaptic inputs from other neurons. Such third signals are known to modulate the amplitude of the backpropagating action potential, and thereby to critically influence the changes of synaptic weights elicited by spike-timing-dependent plasticity (STDP). Furthermore, we examine in this article whether one can derive from IB principles a rule for synaptic plasticity that establishes generic computation in neural circuits: the extraction of temporally stable (slow) sensory stimuli (see, e.g., Wiskott & Sejnowski, 2002).
The extraction of relevant features and the neglect of irrelevant information from given data is a common problem in machine learning, and it is also widely believed to be an essential step for neural processing of sensory input streams. However, which information contained in the input data is to be considered relevant is highly dependent on the context.
In a seminal paper Tishby et al. (1999) proposed an information-theoretic definition of relevance with regard to a given context and also presented a batch algorithm for data compression minimizing the loss of relevant information. This framework, the IB method, is aimed at constructing a simple, compressed representation Y (relevant features, the IB) of the given input data X, which preserves high mutual information with a relevance (or target) signal R, which provides contextual or side information. In the IB framework, the amount of relevant information contained in a random variable is explicitly defined as themutual information of this variable with the relevance signal R. Multiple algorithms rooted in the IB framework have been fruitfully applied to typical machine learning applications such as document clustering, document classification, image classification, and feature extraction for speech recognition (see Harremoes & Tishby, 2007).
Extending recent results, we propose a simple learning rule for the weights of spiking neurons derived from the information bottleneck (IB) framework that minimizes the loss of relevant information transmitted in the output spike train. In the IB framework, relevance of information is defined with respect to contextual information, the latter entering the proposed learning rule as a third factor besides pre- and postsynaptic activities. This renders the theoretically motivated learning rule a plausible model for experimentally observed synaptic plasticity phenomena involving three factors. Furthermore, we show that the proposed IB learning rule allows spiking neurons to learn a predictive code, that is, to extract those parts of their input that are predictive for future input.
Information theory is a powerful theoretical framework with numerous important applications, including in the context of neuroscience, such as the analysis of experimental data. Information theory has also provided rigorous principles for learning in abstract and more biological realistic models of neural networks. Especially the learning objective ofmaximizing information transmission of single neurons and neural networks, a principle often termed InfoMax, has been intensively studied in Linsker (1989), Bell and Sejnowski (1995), Chechik (2003), Toyoizumi, Pfister, Aihara, and Gerstner (2005), and Parra, Beck, and Bell (2009). This learning principle has been shown to be a possible framework for independent component analysis; furthermore, it could successfully explain aspects of synaptic plasticity experimentally observed in neural tissue. However, one limitation of this learning objective for gaining a principled understanding of computational processes in neural systems is that the goal of numerous types of computations is not a maximization of information transmission (e.g., from sensory input neurons to areas in the brain where decision are made).
Rather, a characteristic feature of generic computations (e.g., clustering and classification of data, or sorting a list of elements according to some relation) is that they remove some of the information contained in the input. Similarly, generic learning processes require the removal of some of the information originally available in order to achieve generalization capability.
Tishby, Pereira, and Bialek (1999) created a new information-theoretic framework, the information bottleneck (IB) framework, which focuses on transmitting the maximal amount of relevant information. This approach takes a step toward making computational and learning processes more amenable to information-theoretic analysis. We examine in this article whether the IB framework can foster an understanding of organizational principles behind experimentally verified synaptic plasticity mechanisms that involve a third factor" (Sjostrom & Hausser, 2006; Hee et al., 2007).
These are plasticity effects where the amplitude of the synaptic weight change depends not only on the firing activity of the pre- and postsynaptic neuron, but also on a third signal that is transmitted, for example, in the form of neuromodulators or synaptic inputs from other neurons. Such third signals are known to modulate the amplitude of the backpropagating action potential, and thereby to critically influence the changes of synaptic weights elicited by spike-timing-dependent plasticity (STDP). Furthermore, we examine in this article whether one can derive from IB principles a rule for synaptic plasticity that establishes generic computation in neural circuits: the extraction of temporally stable (slow) sensory stimuli (see, e.g., Wiskott & Sejnowski, 2002).
The extraction of relevant features and the neglect of irrelevant information from given data is a common problem in machine learning, and it is also widely believed to be an essential step for neural processing of sensory input streams. However, which information contained in the input data is to be considered relevant is highly dependent on the context.
In a seminal paper Tishby et al. (1999) proposed an information-theoretic definition of relevance with regard to a given context and also presented a batch algorithm for data compression minimizing the loss of relevant information. This framework, the IB method, is aimed at constructing a simple, compressed representation Y (relevant features, the IB) of the given input data X, which preserves high mutual information with a relevance (or target) signal R, which provides contextual or side information. In the IB framework, the amount of relevant information contained in a random variable is explicitly defined as themutual information of this variable with the relevance signal R. Multiple algorithms rooted in the IB framework have been fruitfully applied to typical machine learning applications such as document clustering, document classification, image classification, and feature extraction for speech recognition (see Harremoes & Tishby, 2007).
Download the book A Spiking Neuron as Information Bottleneck for free or read online
Continue reading on any device:
Last viewed books
Related books
{related-news}
Comments (0)