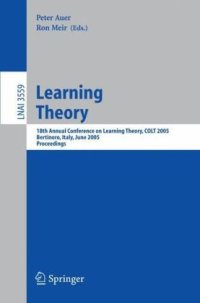
Ebook: Learning Theory: 18th Annual Conference on Learning Theory, COLT 2005, Bertinoro, Italy, June 27-30, 2005. Proceedings
- Genre: Education
- Tags: Computation by Abstract Devices, Algorithm Analysis and Problem Complexity, Mathematical Logic and Formal Languages, Artificial Intelligence (incl. Robotics)
- Series: Lecture Notes in Computer Science 3559 : Lecture Notes in Artificial Intelligence
- Year: 2005
- Publisher: Springer-Verlag Berlin Heidelberg
- Edition: 1
- Language: English
- pdf
This volume contains papers presented at the Eighteenth Annual Conference on Learning Theory (previously known as the Conference on Computational Learning Theory) held in Bertinoro, Italy from June 27 to 30, 2005. The technical program contained 45 papers selected from 120 submissions, 3 open problems selected from among 5 contributed, and 2 invited lectures. The invited lectures were given by Sergiu Hart on “Uncoupled Dynamics and Nash Equilibrium”, and by Satinder Singh on “Rethinking State, Action, and Reward in Reinforcement Learning”. These papers were not included in this volume. The Mark Fulk Award is presented annually for the best paper co-authored by a student. The student selected this year was Hadi Salmasian for the paper titled “The Spectral Method for General Mixture Models” co-authored with Ravindran Kannan and Santosh Vempala. The number of papers submitted to COLT this year was exceptionally high. In addition to the classical COLT topics, we found an increase in the number of submissions related to novel classi?cation scenarios such as ranking. This - crease re?ects a healthy shift towards more structured classi?cation problems, which are becoming increasingly relevant to practitioners.
This book constitutes the refereed proceedings of the 18th Annual Conference on Learning Theory, COLT 2005, held in Bertinoro, Italy in June 2005.
The 45 revised full papers together with three articles on open problems presented were carefully reviewed and selected from a total of 120 submissions. The papers are organized in topical sections on: learning to rank, boosting, unlabeled data, multiclass classification, online learning, support vector machines, kernels and embeddings, inductive inference, unsupervised learning, generalization bounds, query learning, attribute efficiency, compression schemes, economics and game theory, separation results for learning models, and survey and prospects on open problems.